The Future of Artificial Intelligence
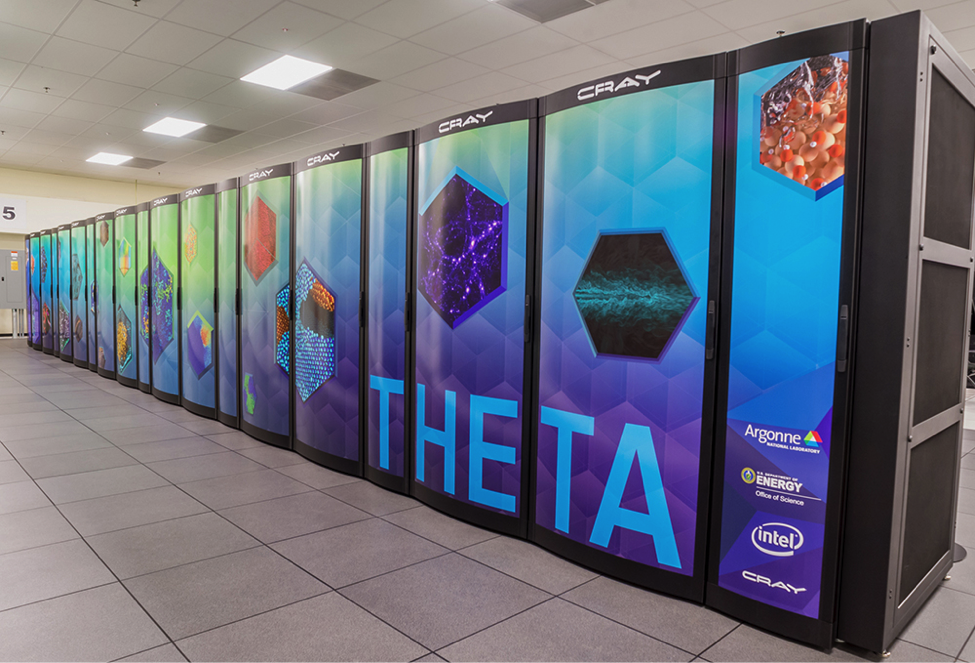
By Xiaoyi Liu
Sci-fi humanoids such as the Terminator or the cyber-agents in The Matrix often come to mind as artificial intelligence moves our cars, gadgets and social networks and in new directions.
But for computing innovator Rick Stevens, associate lab director at Argonne National Laboratory, AI means accelerating fast-thinking computers that could reveal clues to the treatment of diseases such as cancer.
Rick Stevens is associate lab director at Argonne National Laboratory, professor of computer science at the University of Chicago, and principal investigator for the Exascale Computing Project’s (ECP) Cancer Distributed Learning Environment (CANDLE) project. (Image by Argonne National Laboratory.)
Stevens’ exascale computing project would rev up artificial intelligence with data analysis that runs 1,000 times faster than current computing capacity. Artificial intelligence at this level could optimize climate predictions, designs for innovative machines, and diagnosis for brain injuries as well as customizing cancer treatment.
As the principal investigator for the Exascale Cancer Distributed Learning Environment (CANDLE) project, Stevens is using artificial intelligence and the ever-growing cancer-related data to build predictive drug response models. That means speeding up pre-clinical drug screening to find the optimal drugs among millions of possibilities and drive personalized treatments for cancer patients.
The Department of Energy (DOE) national laboratories: Argonne, Oak Ridge, Los Alamos, and Lawrence Livermore, are drawing upon their strengths in high-performance computing (HPC), machine learning and data analytics, coupled with domain strengths at National Cancer Institute and Frederick National Laboratory for Cancer Research to establish the foundations for CANDLE.
In this drug response challenge, Stevens and his team screen cancer cells with different drugs and build a deep learning model from the results of 25 million screenings. The deep learning model is trained to capture the complex, non-linear relationships between the properties of drugs and the properties of the tumors to predict how well the drug will work on that particular tumor.
The idea is that you could predict the response prior to treatment, according to Stevens. “You would have a prediction of which drugs that patient’s tumor most likely to respond to, and then you would presumably start the patient on those drugs,” said Stevens, a University of Chicago professor who specializes in computational biology.
Currently, cancer treatment isn’t done with a predictive model. Oncologists rely on a set of drugs that are the standards in cancer treatment and they typically choose a combination of drugs from among them.
But what combinations work best for a particular patient? If researchers and doctors need to combine three drugs together out of the 100 given drugs, that would mean 500,000 possible combinations.
“Every tumor couldn’t be tried on half a million drug combinations,” explained Stevens.
That’s why entering computational models that can learn what drug combinations have synergy for a particular individual – a situation where the drugs work together more effectively than the simple combination model – is needed. Based on molecular information of the tumor samples, exascale computing holds the potential to sort through all the combinations swiftly to find the best pairings.
“That’s what our models are trying to do, which is to be able to predict the best sets of combinations for a given tumor, based on existing drugs that we know about,” he said. In this way, they can be used to provide treatment recommendations for a given tumor.
Another goal of this project, according to Stevens, is to use these predictive models to drive experiments and develop new drugs. By predicting which compounds are more effective, these computer-based mechanisms can narrow down the possibilities of drug combinations with the best potential for further testing to greatly accelerate cancer research and cancer drug development. Ultimately this kind of technology will be used on patients, Stevens explained, but right now the models are dealing with tumor samples used in lab to collect the large amount of data needed.
The project is not yet in clinical use, and predictions are made based on tumor samples, instead of making predictions involving patients. These models have to be validated before they can be put into large-scale practice, Stevens explained. He and his team are doing a lot of validation experiments, and the models can already predict response.
“We are just trying to make them better,” Stevens said. “If somebody asks me how soon before normal cancer treatment strategies would use deep predictive models, I think it’s few years away.”
Stevens is leading Argonne’s Exascale Computing Initiative that aims to develop exascale computer systems that can complete 1018 operations per second. That’s a million sets of a billion operations per second – artificial intelligence that is 1,000 times faster than current supercomputers. Such computing systems can quickly analyze mountains of data for predictive models critically needed for climate change, for modeling revolutions of galaxies in the universe, for designing drugs, for traumatic brain injury, and for simulating urban transit systems.
Researchers will tackle manufacturing challenges uniquely solved by computer modeling with Argonne’s Theta, a pre-exascale supercomputer built to integrate simulation, data, and learning to drive forward. (Image by Argonne National Laboratory.)
“Climate models are very, very useful,” said Stevens. “But they need a huge amount of computing, and that’s why we have to build an exascale machine.”
By using quantitative methods to simulate the interactions of the important components of the climate system – including the atmosphere, oceans, land surface and ice – climate models can be used to project what will happen under various climate change scenarios.
Daniel Horton, a climatologist in the Department of Earth and Planetary Sciences at Northwestern University, agreed that exascale computing will provide climate simulations that apply to smaller areas, and will help to improve severe weather projections.
One example for this is the projection of thunderstorms, the most frequent phenomena of severe weather globally. In most global climate models, narrow, towering, thunderstorm clouds are simulated via parametrization due to their coarse resolution – a method that approximates the behavior of thunderstorms, but does not resolve to actual physics. Exascale computing will allow the simulation of the physics of individual thunderstorms within the model, and that ideally will allow the researchers to make better projections of thunderstorms and convective precipitation on a wider scale.
“Global warming is changing precipitation,” Horton said. From a theoretical perspective, the atmosphere can hold more water vapor with increasing temperatures. The consequences of this for precipitation, is that it will likely rain less frequently, but when it does, it will rain more, causing greater risks.
“We would like to be able to improve our ability to project these changes in precipitation,” he said. “And so the ability to resolve individual thunderstorms – using exascale computing, should help us in this process of projecting how precipitation might change into the future and where those changes might occur.”
Climate modeling is very similar to other kinds of models in that they all need a lot of computing, and require bigger computers when higher resolutions are needed, according to Stevens. “All these models have in common that, to do a better job of them require more computing capabilities,” he said. “And that’s what behind the exascale computer.”
Exascale computing is structured on the principles of co-design and integration, with three key focus areas in application development, software technology, and hardware and integration. The applications will exploit new hardware innovations and functions, according to Stevens, and some of these next generation features might find their way into personal electronics or computers in the future.
For Stevens, machine learning and AI are strategies for making machines to solve the problems where researchers don’t yet understand the mechanics. For example, researchers may not know how to approach the challenge of designing a new material from first principles, but if they have thousands of examples of the structure of materials and the properties allow, scientists would be better able to learn the function that is needed to get the property they want.
“We don’t actually know how to write down a way to solve those problems, but we might be able to learn from many, many examples, functions that approximate how to solve them,” he explained. “That’s what behind my interest in this.”
Steven’s longstanding endeavor in applying computing to problems in the life sciences originates from his early interest in biological problems and bringing mathematical methods to understanding them. “Biology represents systems that are complex, and they evolve,” he said. “I’ve always been very interested in evolution and the origins of life and how these systems got the way they are.”
“Now we can generate lots of data, we can actually approximate things like the genotype the phenotype relationship that before we had no idea how to write down,” Stevens said. “This idea of learning these really complex functions and then use them to advance research, that’s what I’m interested in.”